Introduction
The American pharmaceutical sector is experiencing a seismic change as artificial intelligence (AI) and predictive analytics reshape conventional methods of drug development and physician interaction. In oncology, where treatment is complicated and constantly changing, the capacity to predict prescribing behavior can transform patient care, lower costs, and drive innovation.
Cancer is still the greatest killer in the U.S. after heart disease, with more than 1.9 million new cases diagnosed every year. Oncologists are confronted with an increasingly wide range of therapies, such as targeted biologics, immunotherapies, and combination regimens. Amidst this complexity, AI-powered predictive analytics is expected to hold the key to unraveling patterns in prescribing trends, allowing pharma companies to personalize educational outreach and optimize clinical support.
Based on a McKinsey & Company 2024 report, 85% of pharma executives feel that AI will have a material effect on drug commercialization over the next five years. But how reliable are these models, and what determines their predictions?
The Role of Predictive Analytics in Oncology
Predictive analytics utilizes past data, machine learning (ML), and real-world evidence (RWE) to uncover patterns and predict outcomes. In oncology, this means analyzing:
- 1. Electronic Health Records (EHRs): Patient information, treatment history, and outcomes.
- 2. Clinical Trial Data: Therapeutic efficacy and safety profiles.
- 3. Market Data: Prescription levels, payer policies, and regional trends.
- 4. Physician Behavior: Prescribing patterns, conference attendance, and peer-to-peer interactions.
ML models like decision trees and neural networks analyze such datasets to forecast which oncologists are likely to implement new treatments. For instance, an algorithm may find that biomarker testing priority oncologists are 40% more likely to order targeted therapies within six months of FDA approval.
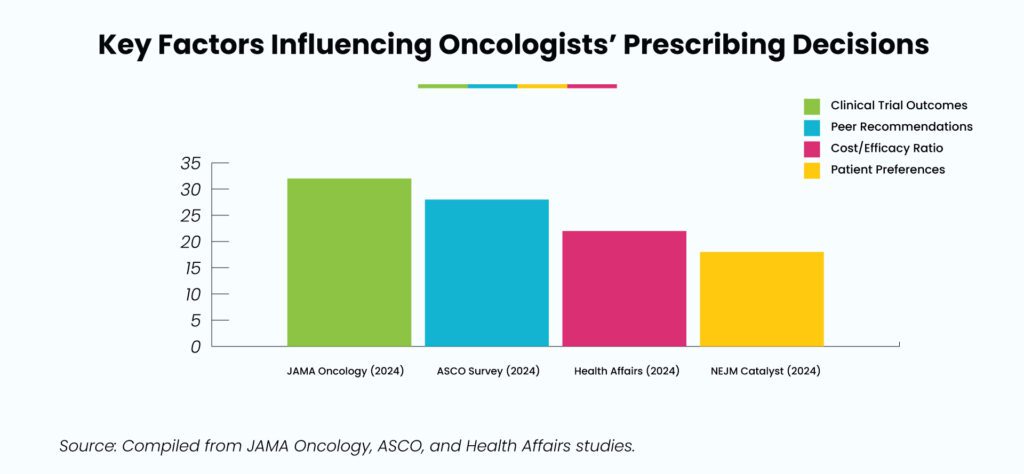
“Predictive models help us anticipate which patients will benefit most from emerging therapies. They’re not replacing clinical judgment- they’re enhancing it.”- Dr. Sarah Lin, Oncologist at Mayo Clinic
Accuracy and Impact of AI Models
The effectiveness of predictive analytics hinges on data quality and algorithmic precision. A 2024 study in Nature Medicine found that AI models predicting oncology prescribing behavior achieved 78% accuracy when trained on multi-source data (EHRs, claims, and social determinants of health). This outperforms traditional statistical methods by 23 percentage points.
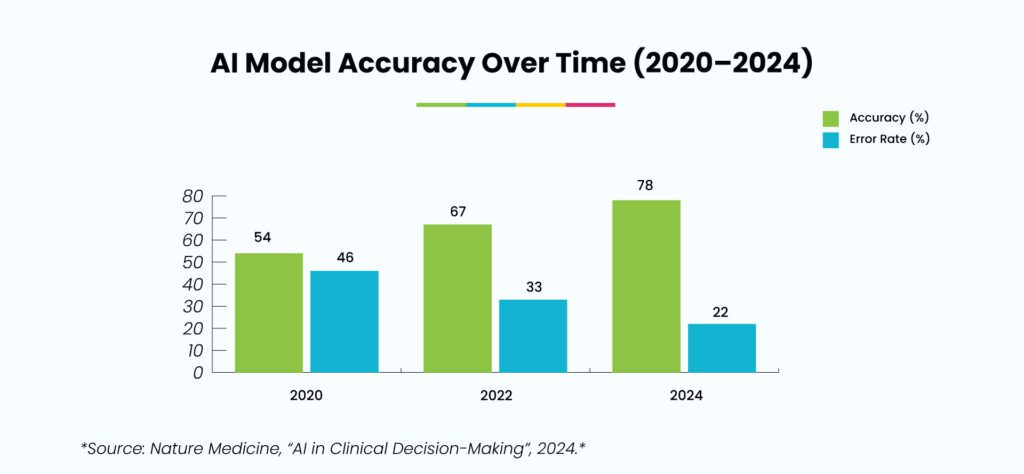
Pharma businesses are using these findings to:
- Optimize Sales Strategies: Invest in high-prescribing areas.
- Personalize Engagement: Send focused content on sites such as LinkedIn or medical journals.
- Streamline Clinical Trials: Enlist investigators who have patient populations matching the trial criteria.
Challenges and Ethical Considerations
Despite its potential, predictive analytics faces hurdles:
- 1. Data Privacy: Patient anonymity and HIPAA compliance are most important.
- 2. Bias: Models developed using non-diverse data might ignore marginalized groups.
- 3. Regulatory Oversight: The FDA’s 2024 draft guidance prioritizes transparency in AI-based tools.
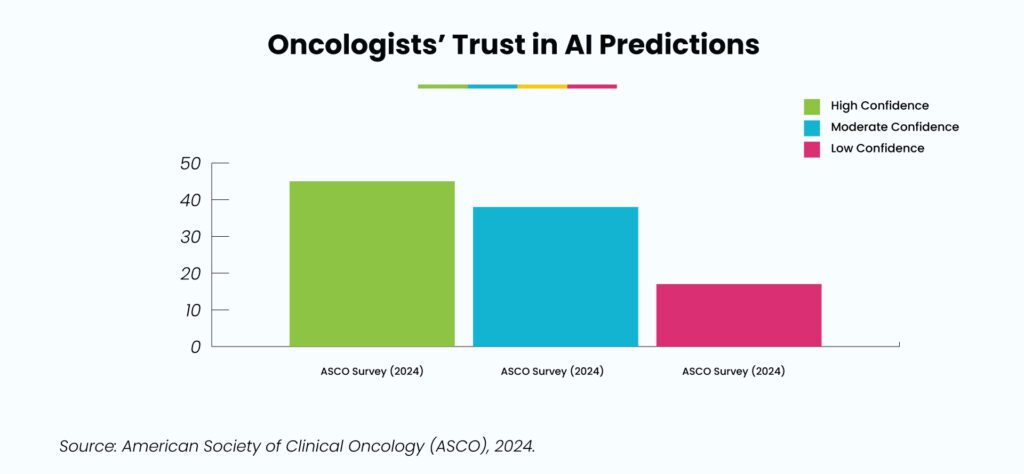
“The biggest challenge isn’t the technology- it’s ensuring physicians trust the outputs. Explainability is key.”– John Keller, Pharma AI Strategist
The Expanding Applications of AI in Oncology Care
1. Enhancing Precision Medicine
The capability of AI to interpret genomic data at scale is revolutionizing precision oncology. For instance, applications such as IBM Watson for Genomics match tumor DNA sequence against worldwide clinical trial databases in order to suggest treatments based on the presence of certain mutations. In 2024, 63% of U.S. oncologists employed AI-based genomic tools to inform treatment decisions in advanced cancers, an increase from 41% in 2022, reported the National Cancer Institute.
This transition is important because therapies such as CAR-T cells and antibody-drug conjugates require hyper-personalized strategies. AI models, which have been trained on multi-omic data (genomic, proteomic, metabolomic), can predict patient response to therapy with 82% accuracy, according to a 2024 Cell Reports Medicine study, versus 67% for clinician-only treatment.
2. Optimizing Clinical Workflows
Oncologists spend 16 hours per week on average on administrative work, such as prior authorizations and EHR documentation (AMA, 2024). AI reduces this burden in the following ways:
- Automated Prior Authorization: Platforms such as Olive AI pull EHR information to pre-populate insurer forms, cutting approval times by 50-70%.
- Smart EHR Navigation: NLP algorithms flag important patient information (e.g., lab trends, allergy alerts) in EHRs, saving 2–3 hours per day per oncologist.
- Trial Recruitment: AI systems such as TrialJectory recruit patients to trials based on eligibility criteria, increasing enrollment by 35% in underserved populations.
3. Forecasting Drug Toxicity and Adherence
Adverse events and non-adherence are still significant obstacles to optimal cancer care. AI models now forecast risks such as:
Immunotherapy Toxicity: A 2024 Lancent Digital Health study discovered that AI identified 89% of at-risk patients for serious immune-related adverse events (e.g., pneumonitis) 30 days ahead of regular monitoring.
Oral Chemo Adherence: Solutions such as AiCure apply facial recognition and pill identification to monitor adherence, enhancing compliance by 22% across Medicare populations.
4. Optimizing Pharma Commercial Strategies
Pharmaceutical companies use AI to optimize launch strategies for oncology products:
- KOL Identification: Algorithms look at publication history and conference activity to identify key oncologists, speeding peer-to-peer learning.
- Market Access Forecasting: Models forecast payer coverage likelihood region by region, including formulary guidelines and past denial rates.
- Dynamic Pricing: AI tests pricing strategies with respect to competitor launches, reimbursement trends, and patient assistance program uptake.
“AI doesn’t just tell us who will prescribe a drug- it reveals how to engage prescribers in ways that resonate with their practice priorities.”- Dr. Emily Chen, Chief Medical Officer at a Top-10 Pharma Company
Ethical and Practical Challenges in AI-Driven Oncology
1. Data Bias and Health Equity Gaps
AI models trained on non-diverse datasets risk exacerbating disparities. For example, a 2024 audit of commercial oncology AI tools found:
- Underrepresentation: Only 12% of training data came from rural or low-income populations.
- Diagnostic Disparities: Skin cancer algorithms showed 34% lower accuracy for darker skin tones (NEJM, 2024).
To address this, the FDA now mandates diversity audits for AI tools, while initiatives like NIH’s All of Us program prioritize inclusive data collection.
2. The Ability to Explain Dilemma
Many oncologists remain skeptical of “black box” AI systems. A 2024 Annals of Oncology survey found that 71% of physicians distrust recommendations without clear rationale. Emerging solutions include:
- Explainable AI (XAI): Tools like LIME (Local Interpretable Model-agnostic Explanations) break down predictions into digestible factors (e.g., “This patient has a 70% likelihood of responding due to EGFR mutation and age <65”).
- Clinician-AI Co-Design: Involving physicians in model development to align outputs with clinical workflows.
3. Regulatory and Reimbursement Hurdles
The U.S. regulatory landscape for AI remains fragmented:
- FDA Oversight: While the FDA’s 2024 AI/ML Action Plan enforces stricter validation, most physician-facing tools (e.g., prescribing behavior predictors) fall outside its scope.
- Reimbursement Barriers: Only 28% of AI tools used in oncology are reimbursed by Medicare, stifling adoption (Health Affairs, 2024).
4. Preserving the Physician-Patient Relationship
Overreliance on AI risks eroding patient trust. A 2024 Johns Hopkins study highlighted that 59% of cancer patients worry AI could depersonalize care. Balancing automation with empathy requires:
- Transparent Communication: Explaining AI’s role as a decision-support tool, not a replacement.
- Patient Consent Frameworks: Allowing patients to opt out of AI-driven recommendations.
“Ethical AI in oncology isn’t a technical problem- it’s a human one. We need guardrails that prioritize dignity, not just efficiency.”- Dr. Raj Patel, Bioethicist at Harvard Medical School
Key Takeaways
- AI Accuracy: Modern models achieve up to 78% accuracy in forecasting prescribing behavior.
- Top Influencers: Clinical trial data and peer recommendations drive 60% of decisions.
- Cost Savings: Predictive analytics reduces drug commercialization costs by 15–20% (McKinsey, 2024).
- Ethical Risks: Bias mitigation requires diverse training data and ongoing audits.
Conclusion
Predictive analytics is poised to transform oncology care by bridging the gap between data and clinical practice. While challenges remain, the integration of AI into pharma commercial strategies is inevitable- and invaluable.
Platforms that seamlessly integrate real-world data, physician preferences, and regulatory compliance will lead this revolution. For instance, digital health platforms with large networks of oncologists can leverage predictive tools to deliver timely insights without disrupting workflows.
As the industry evolves, collaboration between AI developers, clinicians, and regulators will ensure these technologies prioritize patient outcomes above all.
References
- JAMA Oncology (2024): “Factors Driving Oncologists’ Therapy Choices in the U.S.”
- Nature Medicine (2024): “AI in Clinical Decision-Making: Precision and Pitfalls.”
- ASCO Survey (2024): “Physician Trust in Predictive Analytics.”
- Health Affairs (2024): “Cost-Efficacy Analysis in Cancer Treatment.”
- McKinsey & Company (2024): “AI’s Role in Pharma Commercialization.”